US AI Policy Is Stuck in Training Mode
U.S. policymakers have made access to AI compute a top strategic priority, reshaping policies on everything from energy production and export controls to infrastructure planning. But they often approach this priority with training in mind, focusing on the compute needed to develop models, while overlooking inference, the type of compute required to deploy and run them effectively. Given many of the major advances in AI are now happening during inference—not training—this oversight means U.S. policy is not well aligned with the frontier of AI progress and is doing too little to support the adoption and competitiveness of American models.
Training is the initial phase where an AI model learns its general knowledge. It involves pre-training, which forms the base capabilities of a model, and is typically followed by post-training or fine-tuning, which sharpens those capabilities for specific tasks. Inference is the phase where a trained AI model uses this knowledge to respond to user requests. For instance, when a user enters a question or prompt into a chatbot and it generates an answer, that’s inference in action. In short, training equips the model with understanding, while inference is how the model puts that understanding to practical use.
The frontier of AI innovation is shifting from improving training to optimizing inference as many of the most meaningful gains in performance, scalability, and cost efficiency are now increasingly being achieved during inference.
OpenAI’s December 2024 release of the o1 model was the major turning point that showed how optimizing inference can unlock new performance gains. In its release paper, two charts (shown below) compare how much o1’s accuracy improved with more compute during training (left chart) versus inference (right chart). On the left, the model’s accuracy test score rises as more computational resources are invested in training—whether by extending the number of training steps, increasing model size, or combining both—but eventually plateaus, illustrating that merely scaling training hits diminishing returns. On the right, once the model is already trained, giving it more compute at inference, for example, by letting it do multiple “thinking” passes called chain-of-thought steps, drives a sharper boost in accuracy. That’s why o1 tends to take more time to respond to user prompts than earlier models like 4o; it’s designed to “think harder” during inference in order to perform better.
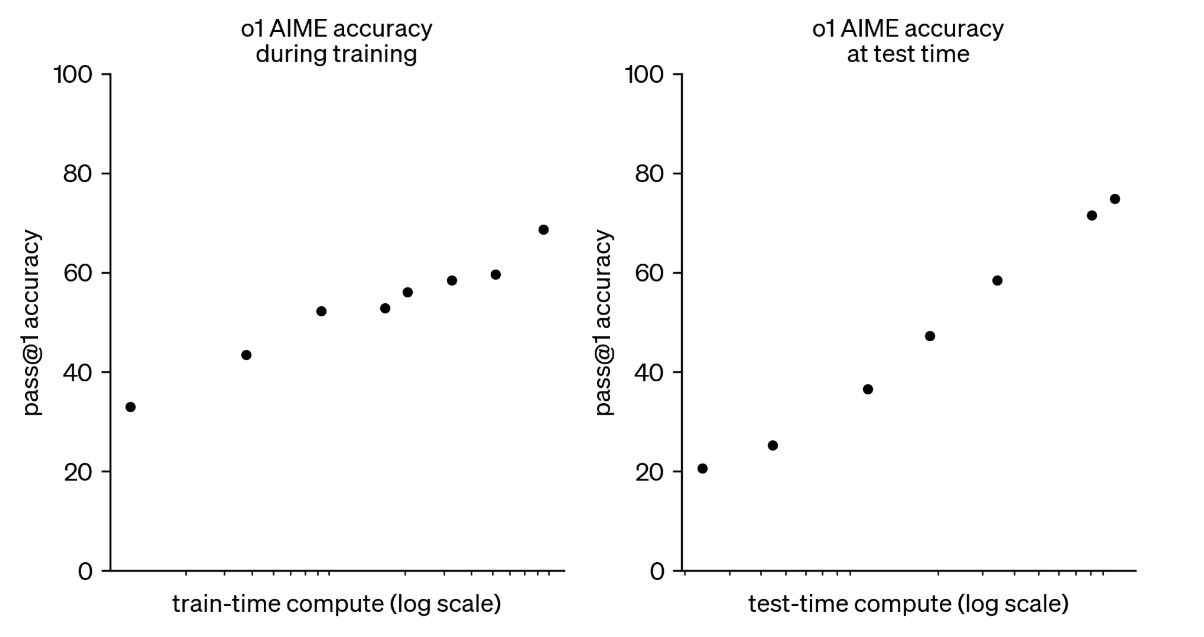
DeepSeek’s January 2025 release of R1, on the other hand, demonstrated that optimizing for inference can deliver major efficiency gains alongside strong performance. A key technique it employs is quantization, which reduces the numerical precision of the model’s calculations during inference. When a user enters a prompt, the model still performs many arithmetic operations to generate a response, but with quantization, those operations use lower-precision data types instead of standard 32-bit floating-point numbers. This significantly cuts the memory and compute required at inference, with minimal loss in accuracy. By reducing bits per calculation, R1 lowers the cost per query—an efficiency that scales profitability and competitiveness dramatically when handling millions or even billions of queries each day.
Despite the growing importance of inference for performance, scalability, and competitiveness, U.S. AI policy remains myopically focused on supporting better training. This oversight is distorting deployment strategies, undermining export controls, and squandering opportunities to boost competitiveness.
Take global deployment. U.S. AI models can only be deployed effectively abroad if there’s infrastructure to support localized inference. Inference needs to happen close to users to reduce latency, cut costs, and ensure reliable service, which is why companies like Microsoft are building data centers in places like Kenya—often called “Silicon Savannah” for its rapidly growing technology sector. While recent domestic policy has focused on building more domestic data centers at home, foreign policy is doing little to support the building of U.S. data centers abroad needed for effective inference. Kenya’s new AI strategy raises concerns over data sovereignty and foreign-controlled infrastructure, while the United States’ abrupt suspension of USAID funding has disrupted the Kenyan economy, damaged trust, and highlighted the risks of dependence in the region. Meanwhile, China continues to position itself as a reliable economic partner, investing heavily in Kenya’s digital infrastructure. U.S. policymakers should recognize building trusted, integrated, and resilient U.S. data infrastructure abroad is a strategic imperative because it ensures global deployment of U.S. models won’t depend on infrastructure shaped by others and supports the adoption of U.S. models over Chinese ones.
Export controls show a similar misalignment. The United States has focused on restricting training-optimized chips like the H100, assuming this would bottleneck China’s AI progress. But as inference becomes the engine of applied AI, China’s access to inference-optimized chips like the H20—which complies with U.S. rules yet outperforms the H100 for certain tasks—shows how the policy misses the mark. Add China’s stockpile of A100-class chips, still highly effective for inference, and it’s clear that training-centric controls are not slowing progress. The entire approach to AI export controls should be reevaluated, but if export policy doesn’t evolve to reflect the shift toward inference, it will leave key loopholes wide open.
Energy policy for AI is no different. The administration has prioritized expanding energy access to support model training, including invoking a national energy emergency to accelerate power generation, while ignoring the competitive advantages of supporting energy-efficient inference. But as is true for the auto industry, governments can make it easier to build powerful engines, but to succeed in a global market, companies also need to sell vehicles that are efficient to operate. Similarly, even if training energy-intensive AI models becomes easier, global adopters will favor models that are less costly to run. That’s part of what makes the energy-efficient models from Mistral, the French AI startup, so competitive. The United States should, therefore, help establish energy transparency standards for AI models, encouraging developers to design more efficient systems and helping buyers choose them.
U.S. AI policy needs to catch up to where the field is today. Recognizing the growing role of inference is a simple shift that could unlock smarter decisions across the board.