
Podcast: The Hype, the Hope, and the Practical Realities of Artificial Intelligence, With Pedro Domingos
There is an inordinate amount of hype and fear around artificial intelligence these days, as a chorus of scholars, luminaries, media, and politicians nervously project that it could soon take our jobs and subjugate or even kills us off. Others are just as fanciful in hoping it is on the verge of solving all our problems. But the truth is AI isn’t nearly as advanced as most people imagine. What is the practical reality of AI today, and how should government approach AI policy to maximize its potential? To parse the hype, the hope, and the path forward for AI, Rob and Jackie sat down recently with Pedro Domingos, emeritus professor of computer science at the University of Washington and author of The Master Algorithm.
Mentioned
- Pedro Domingos, The Master Algorithm: How the Quest for the Ultimate Learning Machine Will Remake Our World (Basic Books, 2015).
- Robert D. Atkinson, “The 2015 ITIF Luddite Award Nominees: The Worst of the Year’s Worst Innovation Killers” (ITIF, December 2015).
- Richard Dawkins, The Selfish Gene (Oxford University Press, 1990).
- Carl Benedikt Frey and Michael A. Osbourne, “The Future of Employment: How Susceptible Are Jobs to Computerisation?” (University of Oxford, September 17, 2013).
- Michael McLaughlin and Daniel Castro, “The Critics Were Wrong: NIST Data Shows the Best Facial Recognition Algorithms Are Neither Racist Nor Sexist” (ITIF, January 2020).
- “The Case for Killer Robots,” ITIF Innovation Files podcast with Robert Marks, August 10, 2020.
Auto-Transcript
Rob Atkinson: Welcome to Innovation Files. I’m Rob Atkinson, founder and president of the Information Technology and Innovation Foundation. We’re a DC based think tank that works on technology policy.
Jackie Whisman: And I’m Jackie Whisman. I handle outreach at ITIF, which I’m proud to say is the world’s top-ranked think tank for science and technology policy.
Rob Atkinson: And this podcast is about the kinds of issues we cover at ITIF, from the broad economics of innovation, to specific policy and regulatory questions about new technologies. And today, we’re talking about one of our favorite new technologies, artificial intelligence.
Jackie Whisman: And our guest is Pedro Domingos, who is a professor of computer science and engineering at the University of Washington, which is the sixth-ranked computer science program in the country. He’s also the author of The Master Algorithm: How the Quest for the Ultimate Learning Machine Will Remake Our World. Thanks for being here, Pedro.
Pedro Domingos: Thanks for having me.
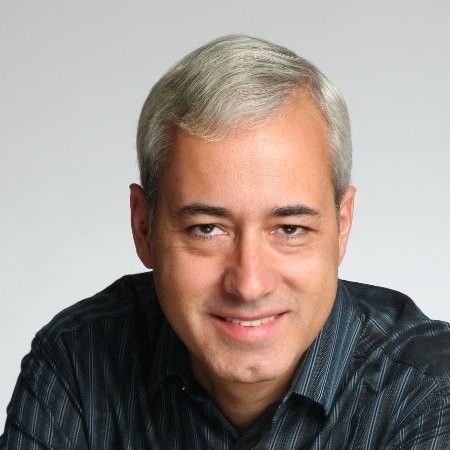
Jackie Whisman: So, one of the big issues that we hear about, both from AI scientists, some of them, but a lot in the media and by politicians are fears around the rise of artificial general intelligence, or AGI. And what are your thoughts on this?
Pedro Domingos: I wouldn’t get carried away with those fears, for two reasons. One is that artificial general intelligence is still very far away. We’ve made a lot of progress in AI, but there’s far, far more still to go. It’s a very long journey. We’ve come a thousand miles, but there’s a million more to go. So, a lot of the talk that we hear as if AI is just around the corner—human level, general intelligence is just around the corner—really is missing a knowledge of the history of AI and knowledge of how hard the problem is. We know now that this is a very hard problem. In the beginning, the pioneers underestimated how hard it was, and people who are new to the field still do that. That’s one aspect. The other aspect is, which is more subtle but ultimately more important, is that even if AGI was around the corner, there’s still no reason to panic.
We can have AI systems that are as intelligent as humans are; in fact, far more, and not have to fear them. People fear AI because when they hear “intelligence” they project onto the machine all these human qualities like emotions and consciousness and the will to power and whatnot, and they think AI will outcompete us as a species. That ain’t how it works. AI is just a very powerful tool, and as long as we... I can imagine hackers trying to create an evil AI and we need a cyber police to do that. But short of that think, for example, that you want to use AI to cure cancer. And this is, of course, a very real application. We want it to be as intelligent as possible, or any other application. So the more intelligent we make the AI, the better off we are, provided that we stay in control.
And there’s a very easy way to stay in control, even if the AI is very intelligent, which is, we control, we determine the objective function that the AI is trying to optimize. Think about a DNA doesn’t understand anything about the plants and animals that it creates; they’re far more intelligent than it is. And yet, the DNA still controls those creatures that it creates. And there’s a more technical aspect to this, which is that AI is all about solving what are called NP-complete problems. Some people even use this as a definition of the field, which are problems that it takes exponential time to solve, but only polynomial time to check. What this means is that you could throw infinite intelligence at solving the problem; all we have to do is check that the solution is what we want, and that is far, far, far easier than actually solving the problem. So, for all these reasons, I think while it’s important to keep an eye on things, and certain people should certainly be thinking about the possible scenarios, the fears around AGI are very overblown.
Rob Atkinson: We used to do this thing that the annual Luddite Award of the year, and it was for people who either were engaged in policy or proposed policy that we thought was just anti-innovation. And one year we gave it to a group of computer scientists, including, I think Elon Musk was in the group, although he’s not a computer scientist. And my favorite thing with Musk is, Musk initially said that AI was going to kill us because it would gain this consciousness and... like the Terminator, but then he walked that significantly back. His latest statement was not that it would kill us; it has no desire to kill us, it would just put us in a guerrilla kind of enclaves up in the mountains where humans would live, and then the AI would live in the cities. And because he’s Elon Musk, people don’t laugh. Don’t like, “What kind of tinfoil hat are you wearing, Mr. Musk?”
And it’s just so easy for people to think that, because I think maybe the problem is they shouldn’t have put intelligence in AI, it just should have been, I don’t know, logic systems or something like that, or... but, anyway.
Pedro Domingos: It was a double-edged sword. It’s like Richard Dawkins calling his book The Selfish Gene, very much a double-edged sword. On the one hand, I think it actually is an appropriate name because it does capture the ambition of the field and brings in a lot of smart people and whatnot. On the other hand, it does have this downside that people just project all these things onto it that just aren’t there.
Rob Atkinson: You mentioned early, early on about the AI and all, and I remember a quote from Marvin Minsky. I think it was the first... the Dartmouth conference that was the famous one, like ‘57 or something like that. And they honestly thought that we’re going to get pretty good AI within three years or two years, and it just goes to show how hard it is.
Pedro Domingos: Yeah. Yeah. There’s this famous story of Marvin Minsky giving to a student as a summer project to basically solve computer vision. There’s a camera, and the computer has to say what’s in the image, and 50 years later we still haven’t solved that problem. So, we do have an appreciation. Again, the paradoxical thing about AI is that we feel it’s easy because evolution spent 500 million years evolving just to do it, but that’s actually extremely hard. In fact, one of the lessons in AI with actually a lot of policy implications is that white collar work is easier for AI than blue collar work. Anything you have to go to college for, by definition, we’re not that good at. It’s actually working in a construction site without tripping over things and whatnot that’s actually really hard.
Rob Atkinson: I like these folks, Osborne and Frey from Oxford. They’re nice guys, but they did this report, I think that you know, and it’s the iconic “47% of jobs will be killed by AI.” And it was really, to me, not a very good scholarly piece of work and partly it was misinterpreted, although they didn’t do enough to stop that. But one of the things I did, when I looked on their list of... it was basically they just generated that by looking at the Department of Labor skills thing or something, and one of the occupations that was going to be replaced by AI was fashion models.
Pedro Domingos: There you go.
Rob Atkinson: And another one was, I put a list of all these occupations. Imagine how AI could do this. I’m having plumbing work done in my house today. I’m sorry; a robot never, ever, ever going to do that. So complex. And there just so many jobs that we have that you just can’t do. I mean, telephone operators? Sure, I get that. Waiters, maybe, in a restaurant delivering your food; sure. Even truck drivers for long haul routes, but the rest of it is very, very hard. So, how do you see that?
Pedro Domingos: There was, as you know, a lot of follow-up to that paper that came to very different conclusions. One of the best, I thought, was... I forget who the authors were now, but they actually decomposed these occupations into the different sub-problems that they involve, which I think is extremely helpful because then what you find is that in almost every job, there are parts that can be done by AI fairly easily, parts that probably will be in the foreseeable future, and parts that are just way beyond the state of the art, and hopefully we’ll get there one day but not any time soon. And this, I think, really is the good perspective to look at things from.
It’s not, “Oh, the jobs are all going to disappear,” or, “there’s nothing to worry about.” In every job in every domain we have to figure out, what are the parts that are best done by machines. And the way you best protect your job from automation is by automating it yourself. You actually want to start using computers to do the parts of your job that could be done by them, because then you’ll do your job much better. So, it’s not “man versus machine”; it’s “man with machine versus man with no machine”, and the men with no machine has no chance.
Rob Atkinson: It’s like the famous chess guy, Kasparov, and he made it clear. He said, “Look, the machine can beat me, but me and a machine can always beat the machine.”
Pedro Domingos: Exactly.
Rob Atkinson: And that’s your point?
Pedro Domingos: Yeah. In fact, in the chess community they call these centaurs. People think that chess playing computers are now the champions of the world, but they’re actually not. The champions of the world are teams of computer and human. And, again, this thing that happened in chess, I think, is going to be the case across the board.
Rob Atkinson: No, exactly, and there are lots of parts of my job that I’m sure it could be routinized. Editing, some of it could be routinized but a lot of it can’t be because there’s judgment calls. I don’t think there’s... no way. I mean, editing for making a mistake with “thats” versus “that’s”, but not the overall thing. I did want to ask you another related question in that. There was a guy, I can’t remember his name, he was a computer scientist, had a nice blog, and he kind of said that the potential of a fourth AI winter, or I guess it would be the third AI winter, is possible. I don’t know. I’d love to hear your thoughts on that.
And the other related point to that is, where a lot of what AI is now is as you’ve written about, is deep learning, but it seems like everybody’s... what’s happening in deep learning, we’re just doing more of it. We’re doing it in different application areas, we’re doing it deeper, but it’s still deep learning; take your datasets, figure them out. Is there something else that we can expect from that, that we don’t have yet, or do we need some kind of new breakthrough? I mean, Gary Marcus was talking about more of, well, we need the next level, and I was just curious what your thought is in that debate.
Pedro Domingos: Yeah. So, to your first question, I don’t think there will be another AI winter for a very simple reason, which is during the previous two or three AI winters, depending on how you count them, there was a lot of hype around AI but there were almost no real applications. So, when the bottom fell out things really went all the way, and at this point that’s just not the case. AI is everywhere. Particularly the larger tech companies, AI is embedded in every aspect of what they do. It’s not just one application. It’s not just search or recommender systems. Everything is using AI, and this is going to spread to other industries and is spreading to other industries. So, there is a certain amount of over-hype, as is inevitable, and that is going to have to die down sooner or later.
Self-driving cars are not as easy as people thought, virtual assistants are not as easy as people thought. And some disappointment is likely to set in, also because when there’s too many claims being made then people... and also, a new technology, when you apply it there will inevitably be some disappointments. But what I think will happen is, if you think about the famous Gartner hype curve, right? I’m an electrical engineer by background and there’s this thing called the gain of an amplifier, and when the gain is very high you can get more than one oscillation. So, it’s going to happen with AI, is that we... and the AI is very much that kind of technology. So, we don’t just see one upswing and downswing, we see multiple ones, but the important thing is the amplitude will die down and the ramp-up is real. So, I think there are going to be some ups and downs, but on balance things are going to get better.
On the deep learning question, I think there’s certainly a lot of the deep learning being done today that is just more applications of the same basic ideas. But I also think there’s a lot of genuine innovation happening. People often say deep learning is just the same ideas from 30 years ago, and there’s some truth to that, but there’s also things like transformers and generative adversarial networks and graph networks that are new. And a lot of innovations that, while they may not be very important to the public, really make it possible to run these algorithms, like backpropagation, in a way that wasn’t before. And there’s so many people doing deep learning research right now, and so many opportunities that I think we’re going to see some more of these innovations that will make a difference.
Having said that, I do think that there’s a ceiling to what you can accomplish with deep learning, or at least with the kind of deep learning that we have today. And we are going to reach that ceiling, and that ceiling is far below AGI. So, I think if you’re in industry, you should think about it in the following way: even if there was no more progress in AI research, I still have a lot of work to do. Just applying the machine learning that is already there, that is going to be transformative, but then there’s the stuff that’s in the labs that it’s going to take 5 or 10 years to come online. But in the long run, the difference is not going to be the deep learning of today; it’s the things that we haven’t invented yet. For all the impact that there has already had, in particular deep learning, the really, really huge impact is in the future, and is with techniques that we don’t have yet. But we know they exist because the brain does them.
Rob Atkinson: Yeah. I had this debate with my son. He was home for Christmas. Well, actually he was home for seven weeks because he could work remotely. And we have this debate, and I said, “Just because the brain can do it doesn’t mean that we can do it.” He’s sort of more on the side of, “Well, if the brain could do it eventually,” and I guess, eventually if... You know, Kane said “the long run.” Maybe 100 years, I don’t know. But to me, going from where we are now to a brain, I’m just curious what your thoughts are on that.
Pedro Domingos: It’s very hard to predict. Anybody who makes a confident prediction about when we will solve this is probably making it up. So, there’s the question of “in principle.” In principle if, like most scientists, you believe that the world is material, whatever you bring best could be written down as a program. So, at that level of in principle, the answer has to be yes. Now, the question is how complex is it? And, therefore, how long will it take us to get there? And there’s people like Ray Kurzweil and many others to say, “Oh, we’re going to solve AI by reverse engineering the brain.” I think that’s completely mistaken. Reverse engineering the brain is a hellishly hard problem. We’ve known the complete circuitry of the C. Elegans worm. It’s only 300 neurons, and we’ve known that for over 20 years; we still have no idea what that simple circuit is doing.
So, I think going that route is just hopeless. But the thing is, the brain is probably, being a product of evolution, is probably way more complex than it actually needs to be. So, I actually think it’s going to be the other way around. We’re going to figure out AI first and then with that, that will actually help us understand what the brain is doing. So, why might it not happen? In principle is one thing and in practice is another. I think the real, and this is indeed the serious problem, is that the brain is just so complex and the intelligence is just so complex that it’s beyond our power. There’s this thing that if the brain was so simple that we could understand it we would be so stupid that we couldn’t.
Right? And I think this has to be taken seriously. It’s a little too easy to say that, because of course we have a worldwide community of brains just trying to understanding one intelligence, so I do believe that eventually we’ll get there, but it’s a really, really steep hill to climb. And I think it’s going to take a lot of inspiration. It’s not going to take one... We’re still in kind of like the Galileo phase of AI, and I don’t think there’s going to be a Newton that solves it. We’re going to need a hundred Newtons, and how long it will take is extremely unpredictable. Maybe... Actually, it is simpler; there is a learning algorithm that is not that complicated that will get us most of the way there with the data that we have and so on. And who... maybe that’ll happen in the next 10 years, but even then it will take a hundred years for that to basically propagate, or it’ll might take a hundred years or it might never happen, so it’s really hard to say.
Rob Atkinson: Yeah. And I guess the other point of that is, as a general rule I think you can never go wrong thinking that technological progress is a good thing.
Pedro Domingos: Yeah, I agree.
Rob Atkinson: So, when we get the problems we’ll figure it out. I’d rather, than worry and panic now.
Pedro Domingos: History shows that technology is for the better, right? We’re much better off now than we were a hundred years ago, or 200 or 1,000. And it’s not by accident; it’s because technologies gives us more power, and AI is a great example of that. And then it all depends on how we use that power, but on balance we wind up using that power for good because we deliberately tamp down on the bad things and build on the good ones. So, I think technology is for the better, but that is not a reason for complacency. It’s for a reason... again, AI is a prime example of this. AI will be for the better, but not because we sit back and assume it’ll be for the better. It’s because we work on making it for the better.
Jackie Whisman: I’d like to talk about AI and bias because we hear a lot about this, of course, and some assert that AI systems are almost all inherently biased, but we at ITIF really don’t see it that way. What are your thoughts on this, including implications for AI policy?
Pedro Domingos: Yeah. I think the notion that AI is inherently biased has been greatly exaggerated. There are these hundreds of papers all claiming that AI is biased in this or that way, and it’s not. Most of them find some correlation between the output of an AI system and race or gender or something like that and then jump to the conclusion that it’s biased, but correlation is not causation. And I could go through any number of these cases and if you understand what’s going on you’ll see that there is no bias. And in fact, far from almost all AI systems being inherently biased, you can actually prove that the great majority of our systems cannot possibly be biased, because they do not know. It’s like, a machine learning algorithm is just a complex formula. y=ax+b does not have biases about race and gender. It can’t. It’s ridiculous to suggest so, but in fact this is what people are suggesting about AI every day. People who don’t understand what’s inside the black box.
I’ve heard people call this the homunculus fallacy. It’s this notion that inside every AI there’s a little homunculus; there’s a man there with all the prejudices of a man, but it isn’t. It’s a kind of anthropomorphization. Also, I think to be honest, I think this belief that AI is inherently biased in some ways, it tells more, it says more about the people who say that than it says about AI. AI is this canvas onto which we project whatever worries us, and whatever ghosts we have. If I’m a libertarian, AI is big brother, which there’s some worries there you find progressive activist, then AI is just full of bias, which again, it’s not like there’s nothing to worry about there, but people just blow these things completely out of proportion.
Rob Atkinson: You know, my favorite example of that; it’s one we just pull our hair out over because it really is one of those things where the media should know better, and that is the NIST study they do. Every year you can submit algorithms. About a hundred algorithms... I guess this was maybe two years ago now; we wrote about it last year... for facial recognition systems, which use AI. And what they found was about, I’m going to guesstimate, 90 out of 100 had some kind of bias, but 10 of them had zero bias. And so the answer to that is, if you’re going to deploy AI systems in the federal government you have to have a rule that says they are those 10, not the 90. End of story, we’re done. They’re not biased. And yet, virtually every single study, every single article about this, says NIST found the algorithm for biased. No, NIST found some were, and the ones that weren’t, and we just say, “Well, you can’t deploy biased AI algorithms.”
Pedro Domingos: I mean, that’s one part of it. And in fact, to build on that this is actually common mistake that people make with AI is, there’s some problem being solved by AI and 9 out of 10 systems fail. To take a completely different example, you probably saw this real tape of these darker robots falling all over themselves, trying to get off a car and open the door and whatnot. And we all had fun with that, but the truth is two or three of those robots actually completed the task. And those are the ones that matter. The 97 bad ones, they are an evolution’s trash heap, right?
And this is exactly an example of that, but also on top of that I think what people call bias needs to be examined very closely. And often when you say that an algorithm is biased, actually it’s not biased. Some things really are biases, but some things that people call biases aren’t biases. So, for example, there have been all these papers about like, “Oh, look at this AI system. It found that programmer is more correlated with man than with woman, so it’s biased.” Well, I’m sorry, don’t blame the messenger. The program isn’t biased, it’s just reflecting reality. So, we also need to be careful about what we mean by bias.
Rob Atkinson: Yeah, absolutely. I worry that this AI bias panic is just going to grow. And again, by no means is it to say you should be allowed to have inherently biased datasets or not take these seriously, but I think the incentives in both academia and in industry are very strong to get this right.
Pedro Domingos: Yeah, exactly. Part of why the problem is so overblown is that it gets a lot of oxygen from a larger reality, which is often what happens with a lot of things, and there’s this very large preoccupation in society with bias and they’re naturally, rightly or wrongly, exaggerated or not. But then academics are part of this environment. There’s this whole field that grew up in the last five years with hundreds of papers being published every year on how to devise AI algorithms, and some of those biases are really there and should be removed. A lot of those biases are not there, and perversely it’s the people who are introducing the biases with their modifications of the algorithms to do bias algorithms that don’t have biases.
Rob Atkinson: Speaking about that, hopefully the next administration can do that.
Jackie Whisman: Yeah. Well, I was going to ask of the final question how you think the Biden administration should approach AI policy.
Pedro Domingos: That is a great question because there’s a lot at stake, and so there’s a lot of things that need to be done. First of all, there’s education. We need to dramatically increase the number of people who are AI literate. Not just the number of AI PhDs, which certainly we could use hundreds of times more, but also just the number of managers and people in every field who know how to use AI for what we do. Even if AI is still largely a black box, AI is going to be a kind of literacy in the same way that computer science, in general, increasingly is a kind of literacy. And I think there’s a lot that the federal government can do to promote that. And then on the research front, there’s a lot of really fundamental progress to make in AI, as we’ve been talking about, and the federal government is the prime funder of such basic research.
So, there should be a lot of funding for AI that is just letting people follow their curiosity, but making sure that it doesn’t all get funneled into one thing, like deep learning. We need to make sure there’s a diversity of directions of research because, unfortunately, the natural tendency of the research community is to do the opposite. So, I think the federal government can play a role in that. There’s also, if you look at what happened with the Cold War and DARPA and whatnot, the federal government is at its best when it has this mission-oriented research projects, like going to the moon. And there’s room for a lot of such projects in AI, and I think, again, the federal government is a great one for doing that.
The next thing is that, and this is why the US in many ways beat the Soviet Union in the Cold War, is that there’s an ecosystem of technology and economics that is much larger than the government, but the government can help seed. And in particular, something that really worries me today is that we don’t see the kind of collaboration between the tech companies and the government that we need to have. We see that more in China, perversely, partly because they have no choice; the government tells them what to do at the end of the day. But also because in the US, unfortunately, there’s this generation of people in the tech companies who think government is evil and think working for defense is evil. You work at Google and you don’t want Google to do defense work, which is ironic because without defense work there would be no internet and no Google. So, we really need that part to be there. And we need the government and the tech companies to help each other in the same way that, unfortunately, China, they already do more of.
The government also has a very important role in monitoring the tech companies and making sure that bad things don’t happen, because it is true that tech companies are very powerful. It is because of network effects and having more that is good so the solution, I don’t think is to break them up; nevertheless, there is a lot of power there and that power could be misused. How do you regulate AI, I think at the end of the day is very different from how you regulate other technologies that... You can’t write down on, even on a 1,000-page bill, how AI will be regulated because AI moves too fast for that. What you have to do is write down, again, certain constraints, certain parameters that the objective functions of the AI have to satisfy that we, as a society, decide, and then the government needs to have its own AIs whose job is to deal with the other AIs. There’s a few sectors where this already happens, like in finance, for example. There’s a lot of AI used for monitoring compliance and whatnot. So, I think the government itself needs to have AI.
And then the final aspect is the military one, which has always been very important in technology. Technology and military applications have always gone hand-in-hand. And again, I think a lot of people have this paranoia that we’re going to have killer robots rampaging through the streets and, therefore, AI in the military should be banned, when in fact I think it’s the exact opposite. First of all, a military without AI, 50 years from now, will have no hope against the military that has AI. That’s number one. But number two, intelligent weapons are a good thing. Intelligent weapons save lives. Every time that you replace a soldier by an AI, you’re actually taking one human being out of harm’s way. You’re also decreasing the risk, if the AI is properly done, that innocent bystanders will be killed, that people will do things in anger, et cetera, et cetera. So, I think instead of trying to ban machines in combat, well, we should try to do is ban humans in combat.
Rob Atkinson: Couldn’t agree more. We had a podcast this summer with Robert Marks, who’s a computer science and engineering professor at Baylor who wrote a book about killer robots, and I 100% agree with you. That’d be great. And the other reason we’re going to need them is China’s going to get them. Russia’s going to get them; they’re working on them, so. Before we close, because we want to wrap up, Pedro; first of all, I loved your comments about what the Biden administration should be doing, what are the main areas. I really like the idea of mission-oriented AI projects. One of my... in my mind should be machine vision. If we can really make real progress in that in the next 5, 10 years, it would open up so much good things. And I’m sure there’s other things and specific that could really be moonshots, like this is the gold. Kind of like the DARPA Grand Challenge with cars. Let’s get a machine vision. Your machine has to be able to do X by this amount, under this lighting circumstances, whatever it might be. It seems to me that would help drive and then put money behind it.
Pedro Domingos: Yeah. I mean, I think one such a moonshot would be to produce a home robot. Because vision, one of the things that we’ve learned is that you cannot really do vision independently of what’s being done for, so the vision... it’s because the computational cost of vision is so immense that you have to decide what to focus on, at the level of attention at a subconscious level. And we have vision in order to manipulate things in the world, and having a robot in every home would be nice, just like having a car in every garage is nice. And in order to do that you need to develop vision and robotics, and you’d have to need to develop vision and robotics that work with each other. It’s a very large project, but it’s a good example of a moonshot that we could take. And not only would it be a good thing in itself, the spinoffs in terms of the vision itself, the robots itself, et cetera, would be enormous.
Rob Atkinson: And the savings, for example, for letting older people stay in their home longer, all these other applications, delivering the mail. Amazing.
Pedro Domingos: Exactly. I mean, such a robot on its own would be a revolution, even without the spinoffs.
Rob Atkinson: You and I both agree on that, and whenever anybody asks me what I think is the most important technology for the next 30 years, I say it’s robotics that can do these kinds of things. I think that impacts would be so enormous, and boy, we’d all be so much better off, so. Well, this is great, Pedro. I feel like we could go on for at least another hour, but our listeners have a 30-minute window, so. So, anyway, thank you so much for being here. It was just great.
Pedro Domingos: Thank you.
Jackie Whisman: And that’s it for this week. If you liked it, please be sure to rate us and subscribe. Feel free to email, show ideas or questions to [email protected]. You can find the show notes and sign up for our weekly email newsletter on our website, itif.org, and follow us on Twitter, Facebook and LinkedIn: @ITIFdc.
Rob Atkinson: And we have more episodes and great guests lined up. New episodes drop every other Monday, so we hope you’ll continue to tune in.